Unlocking the Potential: **Annotation Tools for Machine Learning**
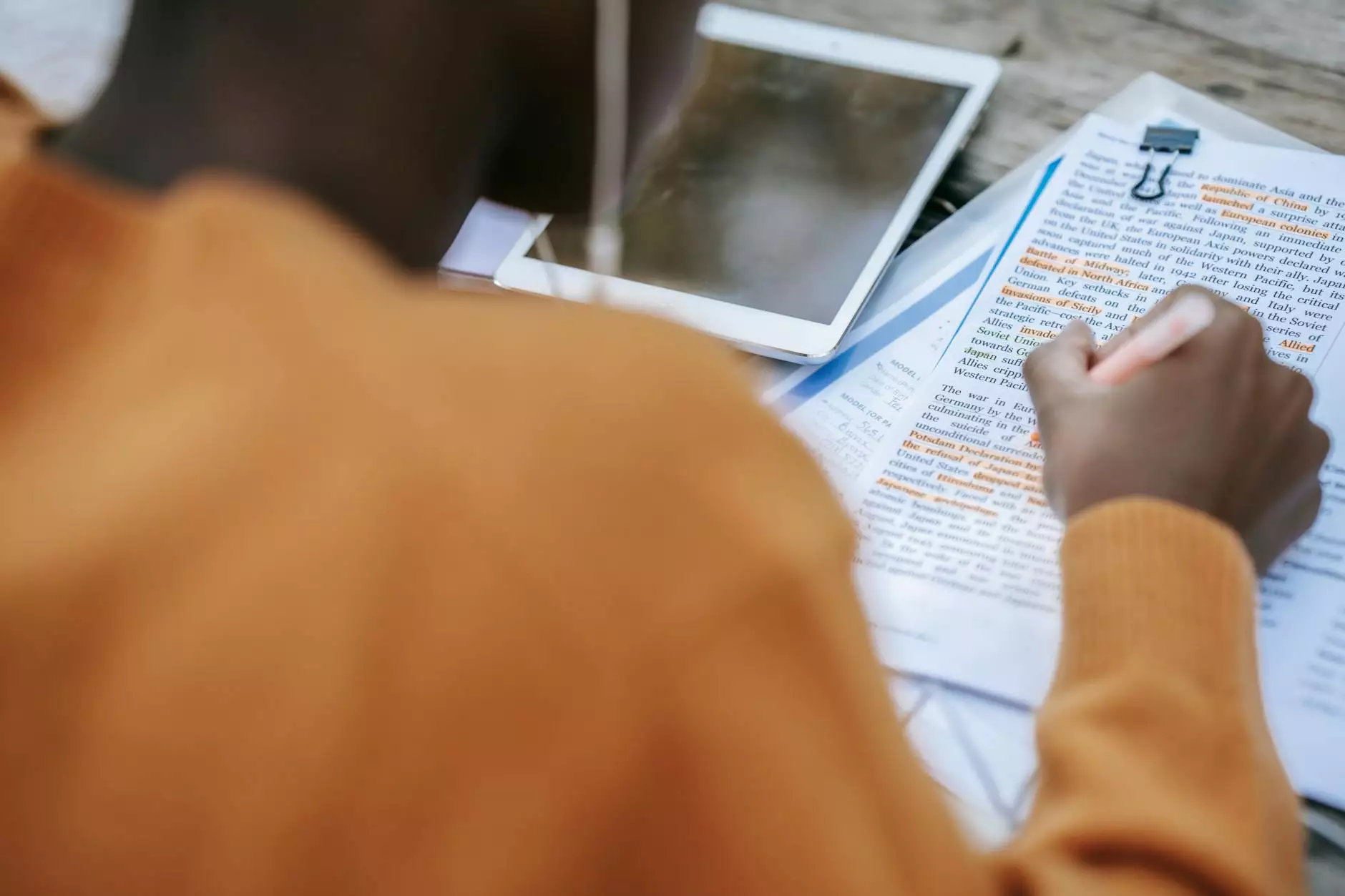
In today’s rapidly evolving technological landscape, the power of machine learning cannot be overstated. Companies and organizations worldwide are leveraging this technology to enhance decision-making processes, optimize operations, and deliver exceptional services. Central to the success of machine learning is the accurate and efficient collection of data, which is where annotation tools for machine learning play a pivotal role. In this article, we will delve into what these tools are, their significance in various fields, and how they contribute to the successful implementation of machine learning projects, particularly in the realms of Home Services and Keys & Locksmiths.
Understanding Annotation Tools
Annotation tools are software applications that enable users to label and categorize data, creating a structured dataset for machine learning models to learn from. These tools support various data types, including text, images, audio, and video. The quality and accuracy of annotated data directly influence the performance of machine learning algorithms, making annotation tools indispensable in the machine learning pipeline.
The Importance of Data Annotation
Data annotation serves as the groundwork for any machine learning model. Here are some key reasons why it is essential:
- Data Quality: High-quality annotated data enhances the accuracy of machine learning models.
- Model Training: Well-annotated datasets ensure that models learn effectively, thereby improving their predictive capabilities.
- Real-World Applications: Accurate annotations allow models to perform better in real-world scenarios, making them reliable for decision-making.
- Scalability: Efficient annotation processes can scale data amounts necessary for advanced machine learning projects.
Types of Annotation Tools for Machine Learning
There are various types of annotation tools specialized for different data formats. Here are several prominent types:
Image Annotation Tools
Image annotation tools help annotate images for tasks such as object detection, segmentation, and classification. Features typically include bounding boxes, polygons, and point annotations. Popular tools include:
- LabelImg: An open-source tool for image labeling.
- VGG Image Annotator: Useful for annotating images with various shapes.
- RectLabel: A paid tool specifically designed for Mac users.
Text Annotation Tools
Text annotation tools assist in labeling textual data for natural language processing tasks. These tools often include features for entity recognition, sentiment analysis, and part-of-speech tagging. Notable tools are:
- Prodigy: A machine learning annotation tool for various text formats.
- LightTag: A collaborative tool for teams working on text data.
- Doccano: An open-source tool for annotating text and training models.
Audio Annotation Tools
Audio annotation tools are designed for labeling sound data for speech recognition, sentiment detection, and sound event classification. Here are a few examples:
- WaveAnnotator: A simple tool for audio file annotation.
- Audacity: While primarily an audio editing tool, it can also be used for annotations.
- Sonix: A cloud-based transcription software with annotation features.
Benefits of Using Annotation Tools
The utilization of annotation tools in machine learning offers numerous benefits. Here are some of the standout advantages:
Increased Efficiency
Automation in the annotation process can drastically reduce the time needed to prepare datasets. Tools can streamline workflows, helping teams focus on model performance rather than data preparation.
Collaboration Capabilities
Many annotation tools provide collaborative features that allow teams to work together in real-time. This is crucial for companies with remote teams or multiple stakeholders involved in the machine learning process.
Cost-Effectiveness
Investing in an annotation tool can save organizations money in the long run by reducing the resources and time needed for data preparation. Automated tools decrease the need for manual labor, thus reducing costs associated with annotation services.
Integration with Machine Learning Frameworks
Most modern annotation tools seamlessly integrate with popular machine learning frameworks such as TensorFlow, PyTorch, and Scikit-learn. This compatibility makes it easier for developers and data scientists to implement their models without significant overhead.
Industry Applications of Annotation Tools
Annotation tools are crucial across various industries, playing a key role in honing machine learning capabilities.
Healthcare
Medical imaging relies heavily on annotated data. For instance, radiologists use annotated scans to train models to diagnose diseases. Accurate annotations can mean quicker diagnoses and improved patient care.
Finance
In finance, annotation tools help in categorizing transactions, detecting fraudulent activities, and understanding market trends through annotated time-series data.
Retail
Retailers use annotation tools to analyze consumer behavior, enabling personalized marketing strategies based on annotated customer data.
Automotive Industry
In the automotive sector, annotation tools assist in developing self-driving cars by annotating images from cameras, helping vehicles recognize and react to objects in real-time.
KeyMakr: Revolutionizing Home Services with Annotation Tools
At KeyMakr, we understand the need for precise and efficient data processing in the realm of Home Services and Keys & Locksmiths. Our commitment to leveraging state-of-the-art annotation tools for machine learning allows us to enhance our services significantly.
Improving Security Solutions
By employing advanced annotation techniques, KeyMakr is able to analyze vast amounts of security footage, identifying potential vulnerabilities and enhancing our locksmith services. This proactive approach enables us to recommend better security solutions tailored to the unique needs of our clients.
Streamlining Operations
Through effective data annotation, we enhance our operational efficiency. By analyzing data on service requests, we can identify patterns and streamline our response times, ensuring that we meet our customers’ needs with maximum efficiency.
Personalized Customer Experience
At KeyMakr, we pride ourselves on delivering a personalized experience to our customers. By using annotated datasets to understand consumer behavior better, we can tailor our services to fit individual needs, creating lasting relationships with our clients.
Future of Annotation Tools in Machine Learning
As technology advances, the future of annotation tools promises even more exciting developments. Here are some anticipated trends:
Enhanced Automation
The integration of artificial intelligence into annotation tools will likely result in further automation, minimizing human errors and expediting the data preparation process.
Improved User Interfaces
With the evolution of user experience design, future annotation tools are expected to adopt more intuitive interfaces, making them accessible to a broader audience.
Real-Time Annotation
As industries demand faster results, real-time annotation tools will become increasingly popular, especially in sectors requiring immediate insights such as finance and security.
Conclusion
In conclusion, the advent of annotation tools for machine learning has fundamentally transformed how organizations approach data analysis and model training. Their significant impact spans across various industries, ensuring that machine learning models reach their maximum potential. For businesses like KeyMakr, these tools not only enhance operational efficiency but also offer personalized services to customers. As we look toward the future, the continuous evolution of these tools will undeniably unlock even more possibilities in the realm of machine learning, providing organizations with the capabilities to innovate and excel in a competitive landscape.